Work
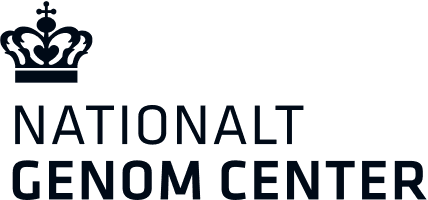
Codellent helped design and implement a quality system for the sequenced genome data, to support the bio-informaticians in handling and controlling the received genome data samples before the samples are sent back to the clinics and stored in a genome database.
During this work Codellent has helped NGC with the data architecture design and platform engineering and data engineering development to support integrations to existing protocols, secure data access and data gateways for the users.
Additionally, Codellent helped implement CI/CD pipelines to secure development speed and deployment quality as well as data models to handle storing, archiving and request of genome-data. For the requests a notification functionality to share status of DNA-analysis in NGC and with the Danish Regions was developed as well.
As strategic advisor and AI implementation partner Codellent has helped our Shipping and Logistics customer with multiple projects around AI and data.
This includes the strategic AI use-case selection and several phases of AI- and Data Platform implementation. Codellent had the responsibility of delivering the cloud AI architecture, setting up infrastructure services, executing on model development, configuring data flows and API-integrations as well as setting up MLOps architecture for re-training and deployment over time.
The AI-models are developed using Deep Learning technology in a Neural Network architecture based several data-sources such as pictures, historical data on measurements and domain knowledge.
With the output from the AI-models the company are able to predict maintenance status and criticality across the world based on data.
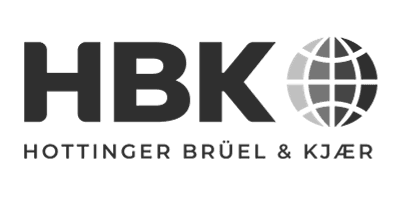
Codellent has helped HBK as advisor and implementation partner on data, strategy, BI and data science.
As HBK are in a major ERP-transformation to SAP S/4, Codellent has helped manage and operate the current data landscape of MS SQL.
In parallel with this Codellent has additionally helped HBK select the future data architecture based on total cost of ownership and cross-functional requirements. The target architecture analysis included technical dependencies, data-on-data insights, organization benchmarks, scenario simulations as well as different end-user needs across domains, areas and countries.
In addition to the to-be architecture Codellent has helped develop and implement the new data strategy covering data products, Data/IT operating model, data ownership processes, business interaction, DevOps, organization and roles.
Currently, Codellent are driving the implementation of a new data platform with Data Products, BI and Analytics.
Codellent has helped design and implement a secure and scalable DevOps framework in which Data Engineers, Data Analysts and business users can utilize data for analysis and decision making.
Based on the DevOps framework the Financial Services company can deliver solid and secure code and data in production while adhering the requirements for compliance and traceability - while still getting performance and value.
During the project Codellent has been responsible for the management of the delivery as well as the design and development of the CI/CD process and features. Multiple technologies such as Python in back-end, SQL in Snowflake, Bitbucket, Bamboo, and DevOps for Orchestration and test combined with Powershell in the implementation.
The project has been successful with additional functionalities being developed "upstream" and "downstream" to implement the usage across different departments and teams.
Articles
In recent years, AI has become the new Klondike, sparking a gold rush that gains momentum with every new trend. One of the latest buzzwords in this AI frenzy is "AI-agent." But what does that even mean? A robotic James Bond?
New tech trends rarely live up to the hype, and the AI vocabulary is already messy and crowded with multiple misconceptions. Adding yet another term like "AI-agent" might seem unnecessary.
Motivated by the promise of AI-agents, this article aims to explore their value, practical use cases, how they work, and how they differ from the equally popular concept of AI-assistants.
In 2024 everyone talks about AI! Everyone - even Her Majesty The Queen - talks about AI as the adoption continues!
However, there is a huge difference between the outspoken perception of what successful AI requires - and the reality of what it takes to succeed with AI. This is also the case in many conversations and companies.
We call this "The AI Iceberg" as metaphor for all the overlooked and undervalued dependencies required to succeed. Creating valuable and long-term success with AI is an ongoing cross-functional exercise requiring...
Most companies have an ambition to utilize AI and run a data-driven business. But what happens when the data quality is insufficient, missing - or maybe even incorrect?
Bad decisions happen leading to risky misconceptions and costly business. Very costly!
Still, data quality seem to be undervalued, overlooked, or framed as a one-time product solution fixed by the data team. To lower cost and improve the potential of data, companies need to understand and align on the shared responsibility of data quality - from management to end-users. This way, by prioritizing and investing in data quality, companies can save cost, improve decision making and remove potential barriers for new innovations with AI...
Career
Connect on LinkedIn